The traiding technologies, such as AI transformation, generate hype. Almost all of the tech-interested people and companies tend to follow the trend and try to catch up with it. But there must be a clear understanding about those technologies, their possibilities, how and where they can be applied. This seems to be crutial to avoid any unnesesary effort, risks and money waisting activities. There is a need to precise the possibilities of AI and especially technologies, AI is scoping under its umbrella.
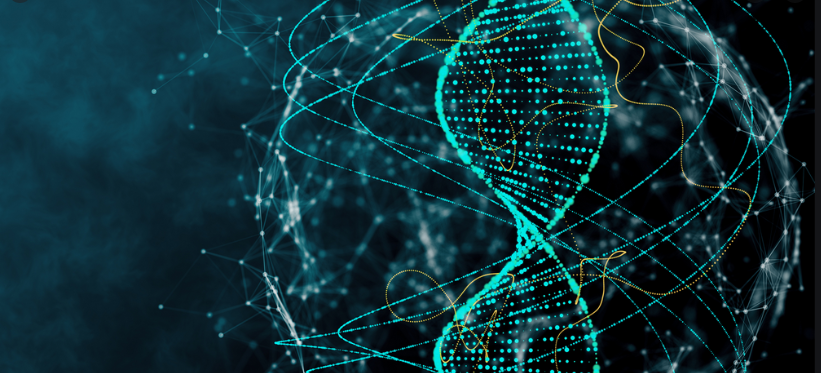
Key factors for AI transformation
Basically it is important to understand that AI is not magic. Of couse AI can be applied to different areas and a lot of issues can be targeted by AI transformation. But Artificial Intelligence and transformational process requires several factors to be taken into account. Artificial Intelligence is very addaptive technology and will vary depending on the data the model is fed with.
Use case
Of course Data and research it is what matters. But creating an use case might be good way to verify the hypothese later down the road. Along with the stakeholders to create a business case, case calculations, hypothesis, associated risks and current technology implementation. Creating an use case at the beginning is a good starting point, but at the end facts, data, feedback and experiment and test results matter more. Those results will either maintain the hypothesis and approve the use case or will reject it totally. Basically those results will be used for definition of the further steps, goals and use cases.
A/B testing has been the most useful method to validate a hypothesis, whether an online or offline campaign. But what is even more important before such testing is to define what success really means to you and to the business. Is it saving X amount of money, or making X amount of sales or providing better customer service?
Data and time
Data… This is most crucial thing about AI. All of the technologies, which summed up under the ambrella of AI, need data. Each and every AI model is only as good as the data it is fed with. The structure of data and analytics in established
organizations could be anything: from having multiple data science teams in each business unit, or to a centralized advanced analytics team at a corporate level. There is no single way of gaining benefits as the structure will depend on the type of industry. Data audit seem definetelly nessesary. Since the data can be changing over a period of time, it is important to ensure the targets are being met and the metrics for success are sustaining data changes. If not, the implementation of a Machine Learning model will not meet the initial purpose. It might make some sense to install some control groups to monitor the Machine Learning model against.
But the another thing is also the time.Since each of the Ai technologies is based on a several learning itterations, those models will take some time to adjust and to be able to provide the user with the proper results.